Termovizijske kamere so cenovno vedno bolj dostopne in tako sem letos ugotovil, da lahko posameznik za razmeroma dostopno ceno nabavi precej zmogljivo termovizijsko kamero. Termovizijska kamera nam omogoča, da lahko vidimo kako tople so površine okrog nas. Toplejše površine oddajajo več sevanja v infrardečem spektru, ki za zaznava senzor v termovizijski kameri.
S tako kamero lahko kvalitetno zaznavamo temperaturi na površini znotraj vidnega polja kamere. Nekdaj bi morali za tako meritev točkovno meriti gosto mrežo točk na površini, zdaj pa lahko zgolj z opazovanjem od daleč v trenutku vidimo temperaturo večje površine.
Čemu služi termovizijska kamera
Kaj se da ugotavljati z napravo, ki zaznava temperaturo?
Vidite lahko področja z višjo in nižjo temperaturo. Če vam okna ali vrata ne tesnijo in je skoznje prepih, se lepo vidi področje, kjer zrak hladi površine. Na okenski polici se vidi tudi hladnejše področje, kar je zaradi konvekcije tudi ob zatesnjenem oknu običajen pojav.
Če radiator ni odzračen ali pa se je v njem nabrala gošča in ne greje enakomerno, se to zelo jasno vidi.
Če se ventila ne da zapreti povsem do konca in nekaj tople vode še vedno kroži skozi ogrevalni sistem, tudi če je razlike samo par stopinj, lahko tak defekten ventil s tako napravo še vedno zaznate.
Če nimate izoliranih toplovodnih napeljav (kar bi bilo edino smiselno) in iztočite nekaj vode, se po steni z časovnim zamikom vidi sled napeljave. Hladno vodo je precej težje videti, ker je razlika v temperaturi manjša in je toplotni tok precej manjši.
Vidi se hladne kote sobe ali toplotne mostove - področja, kjer je zaradi slabe izvedbe izolacije ali konstrukcije pretok toplote večji.
Lahko se vidi tudi konstrukcijske lastnosti stavbe. Na posnetkih na youtube se na ameriških lesenih hišah vidi potek nosilnih stebrov, pri nas pa npr. potek travet - nosilcev montažnega stropa.
Velika večina na električno omrežje priključenih naprav se do neke mere greje. V sobi, ki jo pregledujete s termo kamero, naprave, ki imajo stand-by porabo, kar žarijo.
Če imate večji porabnik električne energije, npr. električni bojler za toplo vodo, se jasno razloči varovalka, preko katere se teče tok.
Če imate električne vodnike, preko katerih teče večji tok, lahko s termovizijsko kamero preverite, da so spoji kakovostni in da ne prihaja do pregrevanja.
Če imate kurišče na polena, lahko preverite če je pepel res ugasnil in ga je varno odložiti.
S termo kamero se lahko vidi tudi izparevanje vode, saj voda ob izparevanju ohlaja površino, s katere izpareva. Tako lahko hladne zaplate na kameri nakazujejo potencialna mesta, kjer je smiselno preverjati za vlago.
Na podoben način lahko vidite, če je oprano perilo že suho.
In seveda najpomembneje: s termo kamero lahko preverite, če sta kava ali čaj že dovolj ohlajena za zaužitje.
Katere lastnosti so pomembne pri termovizijski kameri
Termovizijske kamere imajo enako kot navadne kamere različno ločljivost senzorjev. Najmanj zmogljivi senzorji imajo ločljivost 80x60 pikslov. Pri teh je slika zelo zrnasta in so bolj na nivoju igrače, čeravno drage. Za resnejšo rabo so uporabni senzorji z 160x120 piksli in več. Zmogljivejši so hitro dražji.
Pri zaznavanju je pomembna tudi razločevanja razlike v temperaturi med sosednjimi piksli, ki nam določa kako majhno razliko v temperaturi bo senzor še sposoben zaznati. Manjšo razliko kot je sposoben zaznati senzor, bolj detajlen in dinamičen bo prikaz temperaturnih razlik in manjše razlike v temperaturi bodo potrebne, da opazimo anomalijo.
Vsaka termovizijska kamera ima tudi omejeno temperaturno področje delovanja, ki se tipično nahaja nekje od malo pod minusom in nekaj sto stopinj celzija. Vsekakor se je potrebno zavedati kakšno temperaturno območje je tisto, ki bi ga želeli opazovati.
Pomemben je tudi zorni kot termovizijske kamere. Cenejše kamere imajo nezamenljivo lečo, s katero je določen zorni kot. Za običajno rabo so najbolj uporabne takšne, ki imajo zorni kot nekje med 30° in 50°. Dražje naprave omogočajo menjavo leč, tako da npr. omogočajo manjši zorni kot in je z njimi potem možno snemati tudi z večje razdalje.
Večina cenejših kamer ima omejeno možnost ostrenja slike oz. nastavljanja fokusa.
Ostale stvari so stvar tega kako specializirano opremo želite, koliko dodatnih funkcij in katere, ter koliko vam priložena programska oprema olajša delo. Med dodatnimi funkcijami so pogosto povezljivost preko wifi in upravljanje preko aplikacije na telefonu, snemanje videa, nastavljanje fokusa, zamenjljive leče in tako dalje. Priložena programska oprema pa je tipično predvsem namenjena enostavnejšemu ustvarjanju poročil o pregledu.
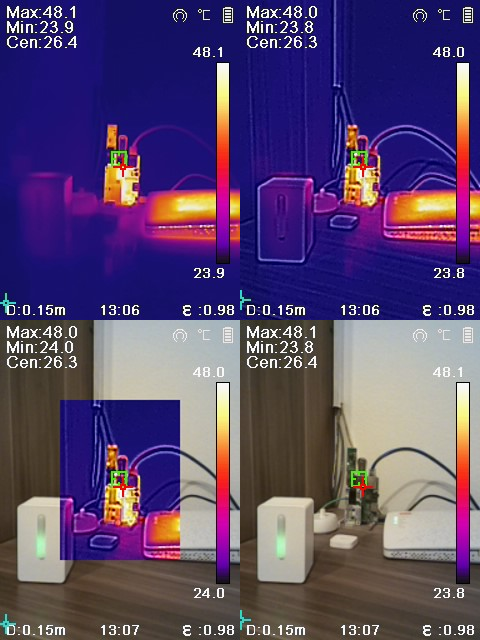
Elektronske naprave v IR spektru žarijo. Primerjava različnih načinov prikaza IR kamere. Levo zgoraj je samo termalna slika. Desno zgoraj je kombinirana slika, ki v enem prikazu združi vidni in IR spekter. Levo spodaj je prikaz slika-v-sliki, desno spodaj je samo vidni spekter.
Triki in namigi
Ker termovizijska kamera zaznava infrardečo svetlobo, je potrebno pri interpretaciji opazovanja nekaj pazljivosti. Različni materiali namreč različno intenzivno sevajo IR svetlobo, kar poimenujemo emisivnost. Posebej je treba biti pazljiv pri kovinah.
Človeška koža ima emisivnost med 0.97 in 0.999, kar pomeni, da izseva praktično vse. Beton in zidak imata emisivnost okrog 0.90. Oksidiran aluminij ima emisivnost 0.20, zloščene kovine pa imajo zelo nizko emisivnost, samo 0.04.
Ker termovizijska kamera izračunava temperaturo površine posredno, na podlagi zaznanih emisij IR svetlobe, bo zaznana temperatura kovinskega dela precej zgrešena.
Podoben pojav je odbojnost. Zelo gladke površine delujejo kot zrcalo in v njih vidimo odsev drugih predmetov.
Če je potrebno odčitavati temperaturo kovinskih predmetov ali predmetov, ki delujejo kot zrcalo, je najlažji način, da jih prelepimo s krep selotejpom. Če tega ne moremo narediti, je potrebno nastaviti emisivnost oz. si predmet ogledati z različnih zornih kotov, da se prepričamo, da ne gre za odsev.
Par posnetkov
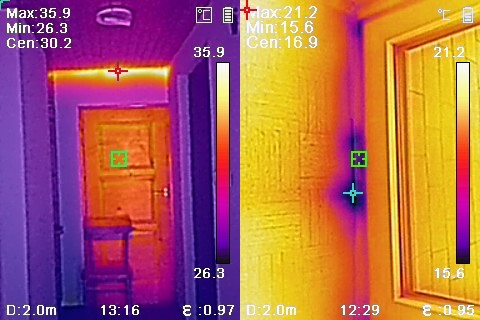
Levo: Neustrezna mansardna izolacija, tram je takoj za montažno steno.
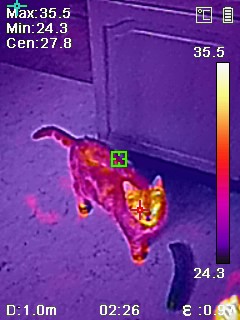
Mačka, ki se ne boji kumare
Ali potrebujete termovizijsko kamero?
Termovizijska kamera je za posameznika še vedno relativno draga stvar, saj osnovne termovizijske kamere stanejo 400 do 500 evrov. Nedvomno pa so cene padle do te mere, da bi tako orodje moral imeti vsak, ki opravlja obrtniško delo, ki vključuje vroča dela, električne napeljave, vodovodne napeljave ali toplotne stroje.
- Viri in sorodno branje:
V Sloveniji se pojavljajo prvi baterijski električni avtobusi. Javno podjetje Marprom, ki skrbi za mestni potniški promet v Mariboru, je objavilo novico, da je vozni park bogatejši za dva nova baterijska električna avtobusa.
Marpromova nova baterijska avtobusa
Nova avtobusa Marproma naj bi se bila zmožna napolniti v 5 minutah, s to energijo pa lahko opravita pet voženj. Avtobusa bosta vozila na liniji 6 - Vzpenjača. Do sedaj so na ti liniji vozili štirje avtobusi, ki so za skupaj 290.000 kilometrov letno pokurili 116.000 litrov dizla. Dva od teh bosta sedaj električna.
Skupna vrednost nakupa avtobusov znaša milijon evrov, poleg dveh avtobusov pa sta bili postavljeni še dve hitri polnilnici, ki sta stali blizu 290.000 EUR.
Gre za avtobusa Iveco E-way dolžine 12 metrov in s kapaciteto do 91 potnikov. Marprom se je odločil za različico z manjšo baterijo kapacitete 73kWh serijsko in 88kWh opcijsko. Baterija je osnovana na anodi iz litijevega titanata, tovrstne baterije pa so zelo primerne za hitro polnjenje, so toplotno zelo obstojne in varne (beri: se ne vžgejo), ter imajo dolgo življenjsko obdobje.
Eno polnjenje zadostuje za 5 voženj, polnjenje pa bo potekalo na t.i. oportunitetni način - ko se avtobus ustavi na postaji, se s polnilnice spusti konektor in med postankom se avtobus polni. Avtobus je sposoben polnjenja z do 450kW, za 73kWh energije pa se ob taki moči pretoči v manj kot 10 minutah. Ni pa pričakovati, da bo polnjenje vedno potekalo s tako visoko močjo.
Marpromovo preizkušanje baterijskih avtobusov
To ni prvi preizkus baterijskega avtobusa, ki ga je izvajal Marprom. Pred nakupom so že izvajali vsaj eno evalvacijo drugih baterijskih avtobusov, ena izmed teh je opisana v diplomskem delu iz logistike, ki je obravnavalo scenarije vpeljave električnega ali hibridnega avtobusa v Javno podjetje Marprom.
V diplomskem delu se razdela primerjava med obstoječim dizelskim avtobusom starejše izdelave, ter novim hibridnim in novim baterijskim. Marprom si je izposodil avtobusa in ju preizkusil na lastnih linijah, ter ugotovil spodnje.
Avtobus | Vrsta pogona | Povprečna poraba dizla | Povprečna poraba elektrike | Strošek energenta (v EUR leta 2017) |
Strošek energenta (v EUR leta 2022) |
Mercedes Citaro | Dizel | 45,34 L/100km | 49,83 EUR/100km | 77,08 EUR/100km | |
Volvo Hybrid | Dizel hibrid | 29,65 L/100km | 32,59 EUR/100km | 50,40 EUR/100km | |
Power Green Bus EV 350 | Električni | 106 kWh/100km | 10,54 EUR/100km | 15,90 EUR/100km |
Za izračun stroška za 2022 je bila uporabljena trenutna regulirana cena dizla 1,7 EUR/L. Za izračun elektriko je bila uporabljena cena 0,15 EUR/kWh, kar je trenutna srednja cena med višjo, manjšo in enotno tarifo za poslovnega uporabnika.
Kljub drastičnim podražitvam energije je v letu 2022 obratovanje električnega avtobusa še vedno 3x do 5x ugodnejše na kilometer.
- Viri:
- Nova avtobusa brez emisij izpušnih plinov in hrupa, marprom.si
- Maribor z današnjim dnem bogatejši za dva električna avtobusa, lokalec.si
- Iveco E-way
- LTO battery
- Vpeljava električnega ali hibridnega avtobusa v Javno podjetje Marprom d.o.o., diplomsko delo
- Bus Electrification: A comparison of capital costs
- Po Kranju bo prihodnje leto vozilo osem e-avtobusov
V prejšnji objavi sem primerjal izkoristek različnih kuhalnikov, kakšna pa je trenutno cena kuhanja z različnimi energenti?
Za primerjavo si je dobro izbrati neko otipljivo mero. Če iz pipe natočimo 1 liter vode, ki ima temperaturo 15°C, in jo zavremo, torej segrejemo na 100°C, je za to potrebna sprememba temperature vode v višini 85 K. Ob specifični toploti vode 4,184 kJ/kg K to pomeni 0,0988 kWh oziroma, če zaokrožimo, 0,1 kWh energije.
Kuhanje z indukcijo
Učinkovitost indukcije je 85%, tako da je potrebna vhodna energija, da zavremo liter vode, 0,1kWh krat 100 deljeno 85, torej 0,117 kWh.
Variabilni del električne energije pri največjem ponudniku stane 0,150295 EUR/kWh. Od tega so omrežnina in prispevki 0,044275 EUR in električna energija 0,106020 EUR/kWh.
Skupni strošek zavretja enega litra vode z indukcijo je 0,0177 EUR.
Plin
Plinski kuhalnik ima učinkovitost 31%. Za zavreti 1 liter vode je potrebna vhodna energija 0,1kWh krat 100 deljeno 31, torej 0,3226 kWh.
Kuhanje s plinom iz plinovoda
Plin se pri Energetiki Ljubljana obračunava po porabi. Omrežnina in prispevki trenutno znašajo 0,04721 EUR/kWh, plin pa 0,08876 EUR/kWh. Skupaj to znaša 0,13596 EUR/kWh.
Skupni strošek zavretja enega litra vode s plinom iz plinovoda je 0,0439 EUR.
Kuhanje s plinom iz jeklenke
Jeklenka stane 29,60 EUR. V eni zeleni jeklenki je 10kg propana, ki ima spodnjo kurilno vrednost 46350 kJ/kg. Preračunano v kilovatne ure je to 128,75 kWh plina na jeklenko. (To pa pomeni tudi, da je v eni jeklenki plina za približno 400 litrov zavrete vode iz začetne temperature 15°C.)
Neto cena plina iz jeklenke je torej 0,2299 EUR/kWh, brez upoštevanja dostave oz. prevoza jeklenke.
Skupni strošek zavretja enega litra vode s plinom iz jeklenke je 0,0742 EUR.
Povzetek
Enak preračun sem opravil še s cenami energentov na 1. januar 2021, rezultati pa so vidni v spodnji tabeli in grafu.
Opazimo lahko, da se je vse podražilo. Zaradi visoke učinkovitosti je kuhanje na elektriko manj občutljivo na podražitve. Plin se je podražil precej, plin iz jeklenke (propan) pa nekoliko manj.
Presenetilo me je kako zelo drag je plin iz jeklenke, več kot 4-kratnik stroška kuhanja na elektriko, in to že pred Ruskim napadom na Ukrajino.
Enostaven način kako varčevati s plinom je električni grelnik za vodo. Ta je podobno učinkovit kot indukcija, poleg tega je cenovno zelo ugoden.
Velja pa upoštevati še vsa ostala priporočila za varčno kuho: zelo pomembna je uporaba pokrovke ter pri plinu kuhanje z ustrezno veliko posodo, da plamen ne sega preko dna posode.
Kuhalnik | Strošek EUR/liter zavrele vode v letu 2021 |
Strošek EUR/liter zavrele vode v letu 2022 |
elektrika - indukcija | 0,0131 EUR | 0,0177 EUR |
plin iz plinovoda | 0,0266 EUR | 0,0439 EUR |
plin iz jeklenke | 0,0571 EUR | 0,0742 EUR |
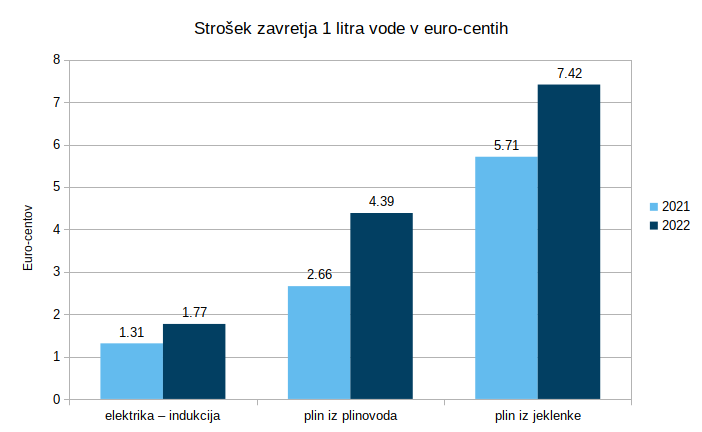
Pomemben pokazatelj razvoja življenjskega standarda je energent za kuhanje. Z višanjem življenjskega standarda se gospodinjstva premikajo od manj čistih energentov pomikajo k bolj čistim in bolj učinkovitim.
Energetska lestev
Ni tako daleč nazaj, ko smo še v Sloveniji kuhali na štedilniku na drva. Naše babice imajo še danes tovrsten štedilnik v hiši. Les je energent, ki je večini naših prednikom omogočil bivanje. Je obnovljiv vir in gorivo, ki ga dobro poznamo, precej dobro pa so poznani že tudi stranski učinki izgorevanja lesa: onesnaževanje s trdnimi delci. Pri kuhanju pa smo s temi onesnaževali še bolj v stiku.
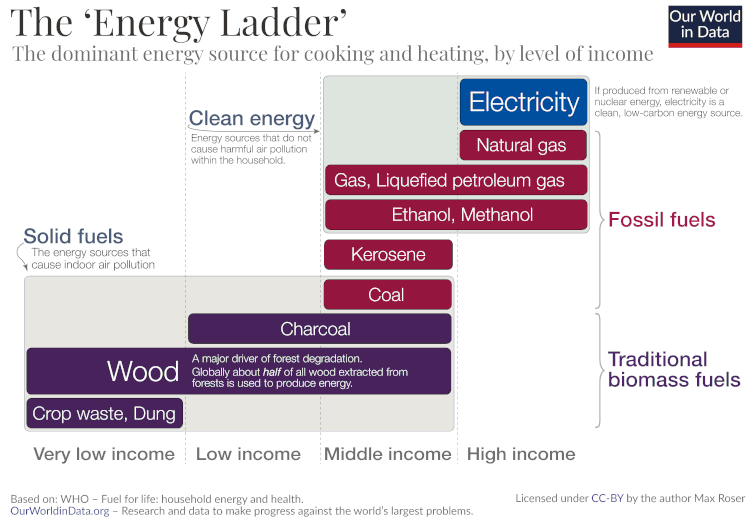
Energetska lestev je poimenovanje za pojav, da se z izboljšanjem življenjskega standarda za kuhanje uporablja čistejši energent. Tako smo z izboljšanjem življenjskega standarda v Sloveniji večinoma prešli na kuhanje na fosilni plin, ki je precej boljši energent. Pri izgorevanju nastaja manj trdnih prašnih delcev, so pa zadnje raziskave pokazale, da tudi izgorevanje fosilnega plina povzroča onesnaževanje zraka v bivalnih prostorih. Ta je povezan s številnimi zdravstvenimi težavami, od astme, do kratkovidnosti, pa še česa.
Z razvojem tehnologije so se pojavili tudi električni kuhalniki. Najenostavnejši električni kuhalnik je navaden uporovni grelec, ki električno energijo pretvarja v toploto, preko fizičnega stika pa toplota prehaja na posodo. Steklokeramični kuhalnik deluje na principu infrardečega sevanja, s katerim se segreva dno posode. Oba ta principa sta "štorasta", saj sta precej neodzivna in se kaj hitro zgodi, da nastaviš previsoko moč, ki je potem ni enostavno zmanjšati.
Indukcija deluje na principu induciranja električnih tokov v dnu posode, ti tokovi pa potem segrevajo kovino, iz katere je posoda. Indukcija odpravlja pomanjkljivost uporovnega in steklokeramičnega grelca in prinaša električnemu kuhalniku odzivnost, ki jo je kuhar vajen pri kuhalniku na fosilni plin.
Učinkovitost naprav
V primerjalnem testu kuhalnikov so ugotavljali učinkovitost kuhalnikov tako da so segrevali dobrih 5 litrov vode. Najhitreje je v 9 minutah vodo segrel indukcijski kuhalnik. Plinski kuhalnik je za segrevanje potreboval kar dvakrat dlje, 18 minut. Električna kuhalna plošča ter steklokeramična plošča sta potrebovali nekaj manj kot plin, sta pa porabili tudi manj energije. Spodnji graf prikazuje temperaturo ogrevane vode v odvisnosti od časa.
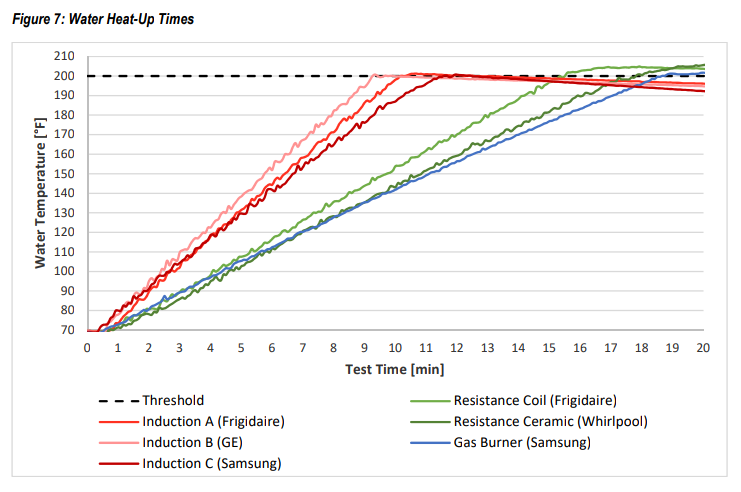
Po primerjanju porabljene energije so ugotovili, da plinski štedilnik dosega učinkovitost okrog 31%, štedilnik z električno ploščo okrog 79%, steklokeramična plošča okrog 75% ter indukcija med 83% in 86%.
Kuhalnik | Učinkovitost |
Plinski gorilnik | 31% |
Električna plošča | 79% |
Steklokeramična plošča | 75% |
Indukcijska plošča | 85% |
Učinkovitost glede na primarno rabo energije
Plin je manj učinkovit, a je za pridobivanje električne energije v termoelektrarnah potrebno sežigati energente, tako da prihaja tudi tam do izgub. Poraja se vprašanje kaj je bolj učinkovito: da posameznik kuri plin v gorilniku ali da kurimo plin v elektrarni, s katero se potem napaja električni kuhalnik.
Spodnja tabela kaže izračun hipotetične učinkovitosti ob upoštevanju, da je elektriko mogoče pridobivati s plinsko turbino z enostavnim ciklom z učinkovitostjo 37%, ali s kombiniranim ciklom z učinkovitostjo 55%. Ob upoštevanju 6% izgub na omrežju je potem izračunana skupna učinkovitost za vsako izmed različic.
Kuhalnik | Plin | Električna plošča | Steklokeramična plošča | Indukcijska plošča | |||
Tip elektrarne | OCGT | CCGT | OCGT | CCGT | OCGT | CCGT | |
Izkoristek elektrarne | 37% | 55% | 37% | 55% | 37% | 55% | |
Izgube na el. omrežju | 94% | 94% | 94% | 94% | 94% | 94% | |
Učinkovitost kuhalnika | 31% | 79% | 79% | 75% | 75% | 85% | 85% |
Skupna učinkovitost | 31.0% | 26.1% | 38.8% | 27.5% | 40.8% | 29.6% | 43.9% |
Prihranek plina z uporabo elektrike |
0.0% | -15.9% | 25.1% | -11.4% | 31.8% | -4.6% | 41.8% |
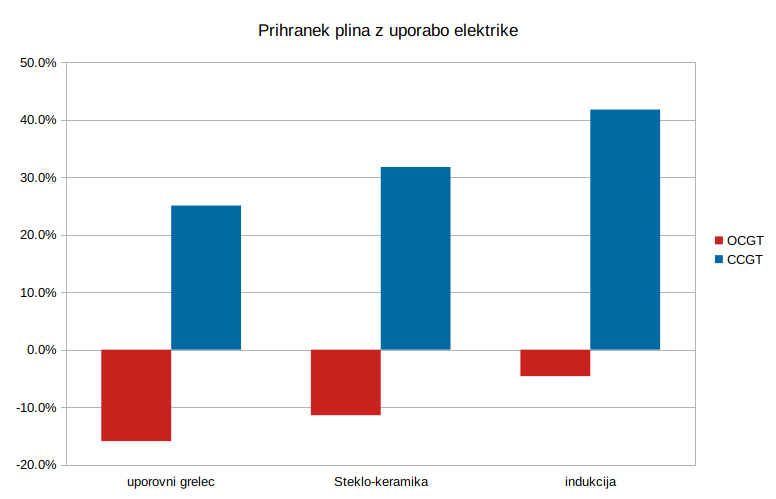
Kuhalnik na plin je z 31% izkoriščenosti relativno neučinkovit. Vidimo, da so električni kuhalniki pri elektrarni na plin vedno manj učinkoviti, če je elektrarna enostavnega cikla, blizu učinkovitosti neposredne rabe plina pride le indukcija. Lahko pa so električni kuhalniki znatno bolj učinkoviti, če je elektrarna kombiniranega cikla z visokim izkoristkom, v tem primeru so vsi električni kuhalniki znatno bolj učinkoviti od plinskega gorilnika.
Ima pa seveda kuhalnik na elektriko še dobro eno dodatno lastnost, in sicer, da znamo elektriko pridobivati na več načinov, ne samo s sežiganjem plina.
Druge težave s kuhanjem na plin
Zdravstvene težave
Pri vsakem kuhanju hrane nastane nekaj onesnaževal, ko hrana pride v stik z vročino. Vendar pa že zaradi samega zgorevanja plina nastajajo fini prašni delci PM2.5, ki so precej strupeni in povzročajo dodatne obremenitve dihal. Poleg prašnih delcev nastajajo še ogljikov monoksid, dušikovi oksidi in formaldehid.
Ogljikov monoksid je nevaren, ker nima vonja, a je strupen in hkrati povzroča zaspanost. Posebej nevarni so primeri, ko pride do zastrupitve z monoksidom med spanjem. Zaradi nezmožnosti zaznave s človeškimi čuti so poleg kurišč obvezni detektorji monoksida. K sreči so zastrupitve z monoksidom relativno redke, saj je za njih potrebno nepopolno zgorevanje, kar pa se vseeno lahko zgodi v slabo prezračenem prostoru, kjer že dlje časa gori plin.
Se pa kaže, da ogljikov monoksid poslabšuje težave pri srčno-žilnih bolnikih.
Dušikov dioksid (NO₂) povzroča težave z dihali in poslabšuje astmo, še posebej pri otrocih. NO₂ pa se tudi povezuje s srčno-žilnimi težavami, diabetesom, s težavami pri porodu, s prezgodnjo smrtjo in rakom. Slabše je tudi delovanje možganov, zlasti pri otrocih. Raziskave so pokazale, da imajo otroci v domovih, kjer uporabljajo plinski štedilnik, kar 42 odstotkov večje tveganje, da dobijo simptome astme.
Plinski kuhalnik skrajša življenjsko dobo za nekje med 0 in enim letom. Pred onesnaževanjem lahko precej pomaga prezračevanje. Če imate napo, ki odvaja zrak ven iz prostora, jo vsekakor uporabljajte, saj znatno in merljivo zmanjša onesnaževanje bivalnih prostorov.
Uvozna odvisnost
V Sloveniji uvažamo plin. Zaradi vojne v Ukrajini je cena plina (zaenkrat) zrastla na trikratnik cene pred vojno. Imamo sicer nekaj zalog v Prekmurju, a jih zaenkrat ne izkoriščamo, saj bi to pomenilo uporabo frackinga. Tudi če bi te zaloge izkoriščali, je to plin, za katerega je država že podelila koncesijo, plin pa se na trgu proda najvišjemu ponudniku, po tržni ceni.
(Ne)zamenljivost energentov
Plin uporabljamo v nekaterih industrijskih postopkih, ki ne morejo plina enostavno zamenjati s čim drugim. Doseganje res visokih temperatur je z elektriko precej težje, tako da je tam plin praktično nujen. Z zmanjšanjem rabe plina v gospodinjstvu ostaja več plina na trgu na voljo za industrijo.
Globalno segrevanje
Zemeljski plin je fosilno gorivo, kar pomeni, da sežiganje tega goriva prispeva k višanju atmosferskega CO₂. Poglavitna sestavina zemeljskega plina je metan, ki je tudi sam toplogredni plin, gledano skozi 20-letno obdobje je toplogredni učinek metana kar 80-krat močnejši kot CO₂. Že res majhno puščanje pri transportu plina lahko hitro postane prevladujoč vir toplogrednega učinka.
Izkazalo pa se je, da vsak plinski gorilnik izpušča nekaj plina. Nekaj plina ne izgori, nekaj ga uide pri prižiganju oz. ugašanju, precej pa ga uide tudi ko je plinski gorilnik ugasnjen.
Vročina
Plinski štedilnik precej segreje kuhinjo, kar bo dobro vedel vsak, ki ga je že kdaj uporabljal na vroč poletni dan. Vsa ta energija, ki gre v segrevanje prostora, pa ne gre v segrevanje posode.
Povzetek
Ali bi morali kuhati na elektriko?
Po mojem ja. Kuhanje z elektriko ima pred plinom kar nekaj prednosti, zdravstvenih, ekoloških in pri udobju bivanja, ko ni več tako vroče poleg štedilnika. Ne glede na kuhalnik močno priporočam uporabo nape, saj precej pripomore k boljši kakovosti zraka.
Pri elektriki je edina smiselna izbira danes indukcija. Indukcijska plošča z odzivnostjo odpravlja še tisto najbolj perečo težavo preostalih različic električnih kuhalnikov. Pred ostalima električnima ploščama ima še dodatno prednost, da kuhalna površina ne ostaja vroča. Za razliko od plina, uporovne električne kuhalne plošče ter steklokeramične plošče se pri indukciji segreva zgolj posoda. Ko posodo odstranimo, se površina, na kateri je bila posoda, ohladi precej hitreje, saj pod njo ni nič vročega.
Cenovno gledano ni enoznačno. Po trenutnih cenah je sicer kuhanje na plin ena-in-pol do dvakrat dražje od kuhanja na indukcijo, a se podražitve pri energentih še niso ustalile. Jasno pa je, da pri kuhanju s plinom ostajamo uvozno odvisni in izpostavljeni podražitvam plina in volji največjih dobaviteljev plina.
- Viri:
- Energetska lestev
- Residential Cooktop Performance and Energy Comparison Study, Frontier Energy Report
- Gas stoves can generate unsafe levels of indoor air pollution, Vox.com
- Your gas stove is always polluting, even when it’s turned off, Vox.com
- Gas stoves are creating unsafe levels of indoor air pollution, OEC Online
- My best estimate is that gas stoves decrease life expectancy by 53 days on average
Spomladanski prazniki so z vidika elektro energetskega omrežja poseben čas. Za veliko noč in za prvomajske praznike je praviloma potreba po električni energiji najmanjša v letu, saj je to ravno čas, ko dovolj upade potreba po ogrevanju in hkrati še ni tako vroče, da bi bilo potrebno za hlajenje poganjati klime.
Nizek odjem pa povzroča tudi povečanje deleža obnovljivih virov energije, oz. povedano drugače - to je čas, ko elektroenergetska omrežja dosegajo rekorde v deležu električne energije, ki jo pridobivajo iz obnovljivih virov, ki je za proizvodnjo najcenejša, saj za delovanje ne potrebuje energenta.
Avstralija kot vodilna država
Avstralija je zelo poveden primer na tem področju. V letu 2019 je v Avstraliji sončna energija predstavljala 6 odstotkov, vetrna pa 7 odstotkov. V letu 2021 se je delež sončne energije podvojil na 12 odstotkov, veter pa je zrastel na 10 odstotkov. Vse to kljub obnovljivim virom nenaklonjeni politični oblasti, ki z obnovljivimi viri energije niti nima nekih podnebnih ciljev, ampak se to tam enostavno finančno izplača.
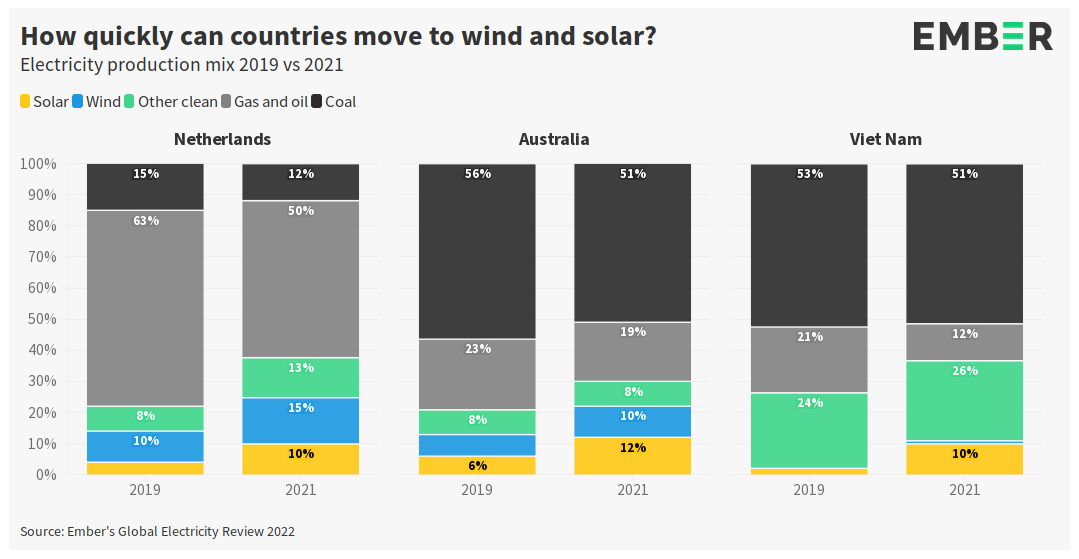
Ti deleži predstavljajo proizvodnjo električne energije na letni ravni. Na dnevni ravni pa to pomeni, da zaradi sončne energije obstajajo velike razlike med proizvodnjo energije med različnimi urami v dnevu. Dostopnost poceni električne energije iz sonca pa precej spreminja tudi porazdelitev cene električne energije skozi dan, kar kaže primerjava cen po urah med zadnjim četrtletjem 2020 in 2021, na kateri se vidi znižanje cen sredi dneva in povišanje večernih in nočnih cen.
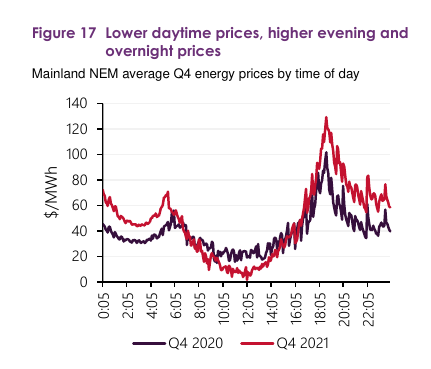
Novi rekordi po svetu
Celinska Avstralija je razdeljena na več med seboj povezanih omrežij, ki so omejene na posamezne zvezne države. Južna Avstralija je zvezna država z manj prebivalci, zaradi naravnih danosti pa dosega zelo visoke deleže obnovljivih virov energije. Tako so obnovljivi viri čez veliko noč dosegli kar 136% delež porabe. Višek nad 100% so seveda izvozili v sosednje zvezne države.
Viktorija, sosednja zvezna država je dosegla 83% delež vetra in sonca. Delež pa bi lahko bil kar 99%, če ne bi nekateri proizvajalci zaradi negativnih cen energije zniževali proizvodnje.
Na drugi strani sveta, v Kaliforniji, je operater prenosnega omrežja CAISO dosegel 97% delež električne energije iz obnovljivih virov energije.
Rekordi pri sosedih
Hrvaški operater prenosnega omrežja HOPS objavlja mesečna in letna poročila o proizvodnji električne energije iz vetrnih elektrarn. V letu 2021 je na Hrvaškem vetrna energija v začetku aprila dosegla rekordni 52,2% delež proizvodnje elektrike glede na odjem.
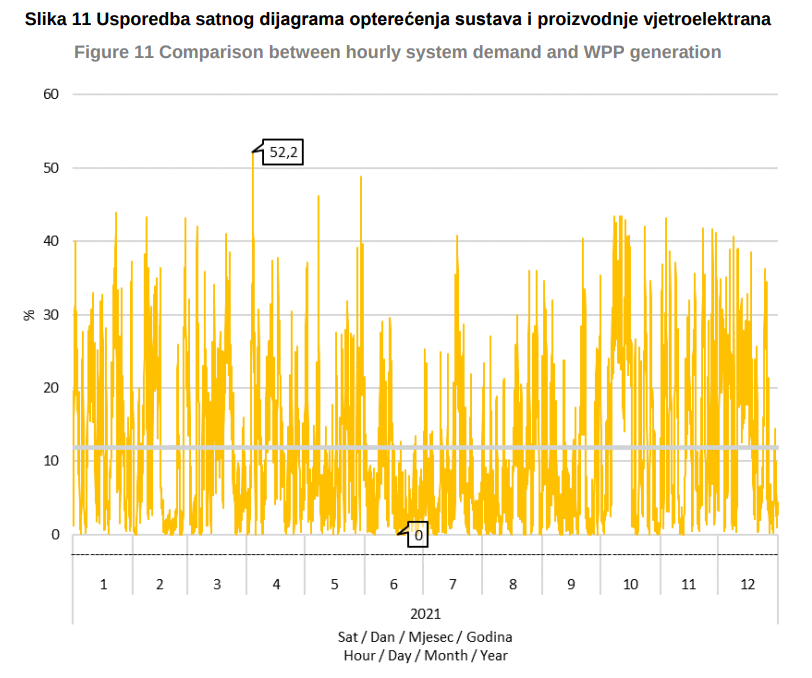
Novi slovenski rekordi?
Najnižji dnevni odjem je bil v Sloveniji leta 2018 1. maja, leta 2019 pa 2. maja. Oboje sovpada s kolektivnimi dopusti dela industrije, tudi zaradi industrije pa je pri nas večino časa še vedno res, da je cena električne energije čez vikend cenejša.
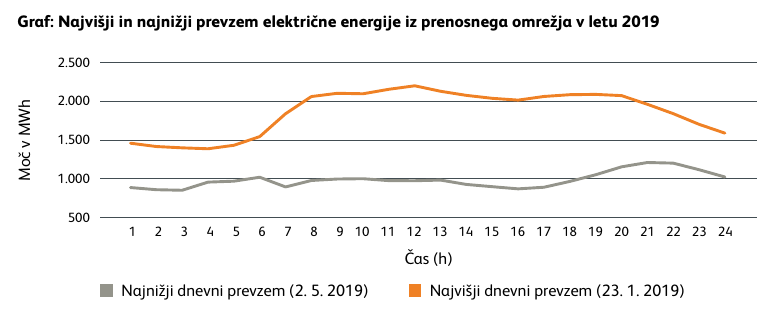
V Sloveniji še nimamo toliko nameščenih sončnih ali vetrnih elektrarn, jih pa imajo druge države, ki so del sinhroniziranega evropskega elektroenergetskega omrežja. Te proizvodne enote pa že pomembno vplivajo na ceno električne energije tudi pri nas. Tako je čez letošnje velikonočne praznike cena električne energije na debelo padla na 0 EUR za MWh.
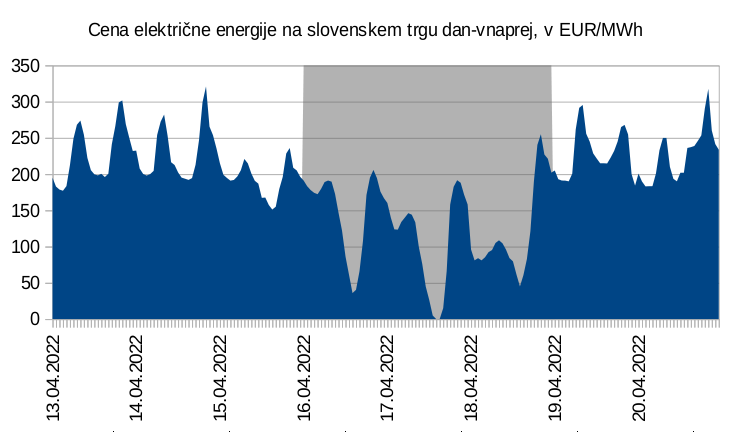
To se pri nas zaenkrat še ne dogaja pogosto. Ob povečevanju količine nameščenih sončnih elektrarn pa bo cena električne energije ob sončnem dnevu tudi pri nas redno znatno nižja kot ponoči, tako kot se to že dogaja v Avstraliji.
- Viri:
- Global Electricity Review 2022, EMBER
- Quarterly Energy Dynamics Q4 2021, AEMO
- South Australia grid reaches record high of 136.6 pct renewables
- Victoria reaches record wind and solar share of 83.8 per cent
- "Re-imagining the grid:"" California reaches record 97.6% renewable share
- Izvještaji o proizvodnji VE, HOPS
- Letno poročilo ELES za leto 2019
- ENTSO-E transparency portal
"Juice - How electricity explains the world" je precej dober dokumentarec o električni energiji iz leta 2019.
Vredno ogleda. Uradna stran in IMDb. Sam sem si ga ogledal na Curiosity Stream, ampak je potreben tudi VPN, ker v Sloveniji ni dostopen.
Dokumentarec se ne ukvarja toliko s tehničnimi detajli, bolj se trudi pojasnjevati kaj električna energija družbi omogoča. Je eden redkih dokumentarcev, ki zelo dobro ilustrira kakšno razliko prinese zanesljivost dobave električne energije. Čeprav nam je v razviti družbi samoumevno, da premaknemo stikalo in se pojavi svetloba, premagovanje teme nikakor ni samoumevno in že svetloba takrat, ko si jo zaželimo, omogoča človeku, da razvija svoje potenciale. Velja pa tudi obratno - nezmožnost osvetlitve bivališča duši človeški potencial.
To pokaže na kar nekaj primerih. V afriški Gani pokaže kako je rastel delež prebivalcev, ki ima dostop do električnega omrežja, in kaj je to pomenilo za ljudi. V Libanonu, kjer državno električno omrežje deluje samo občasno, nekaj ur na dan, pokaže kaj pomeni imeti nezanesljivo oskrbo z električno energijo in koliko stane, ko državno omrežje ne dobavlja energije in je treba poiskati alternativne vire. V Indiji se posamezniki na črno prikapljajo na električno omrežje, tako da imajo operaterji tudi do 40% izgub na omrežju. Kako hitro lahko razviti svet pade v stanje nerazvitega pa je enostavno videti na primeru naravnih nesreč, npr. hurikana Maria, ki je opustošil Puerto Rico, ki si v času snemanja še vedno ni opomogel.
Izpostavi pa tudi nekatere načine kako energetsko razviti svet troši zanesljivo električno energijo, tudi iz fosilnih virov, za določene prestižne dobrine, na primer - gojenje marihuane in kriptovalute.
Dokumentarec se konča z občudovanjem jedrske energije, kar morda nekaterim ne bo všeč. Fizikalno dejstvo je, da je jedrska energija milijonkrat bolj gosta kot fosilni viri glede na maso porabljenega goriva. Vedno mi bo fascinantno, da smo sposobni relativno nadzorovano izrabljati to energijo, nedvomno pa je, da zaenkrat izrabljeno jedrsko gorivo predstavlja velik in nerešen izziv za trajno rešitev. Hramba na dvorišču jedrske elektrarne se mi vseeno ne zdi rešitev.
"Temna stran zelenih energij" oz. angleško "The Dark Side of Green Energies" je "dokumentarec", ki naj bi pojasnil, da pri zeleni energiji ni vse tako čisto kot se zdi.
Na kratko: Ni vredno ogleda, to ni dokumentarec.
Od filma sem pričakoval več. Je nenatančno in slabo artikulirana zmes željá po enostavnih rešitvah, kjer teh ni. V dokumentarcu je kar nekaj napačnih trditev, npr. ta, da so t.i. "redke zemlje" redke. V resnici so v zemljini skorji precej pogoste. Ali pa da so redke kovine "neznane snovi".
Skozi film se vzpostavlja pripoved, da so nekatera področja žrtvovana, predvsem v okoljskem smislu, za zeleno energijo. Obenem se povsem ignorira, da že obstajajo "žrtvovana področja" pri vsem kar človek počne zadnjih par tisoč let. Samo pri nas imamo npr. Idrijo, ki se poseda zaradi rudnika živega srebra. Vas Družmirje ima danes 0 prebivalcev, ker je na njenem mestu zaradi izkopa premoga iz rudnika lignita Velenje nastalo Družmirsko jezero. Rudnik urana v zapiranju Žirovski vrh zahteva stalni monitoring, da radioaktiven material ne pride v Soro in naprej v Savo. V Celju imamo Cinkarno, ki je zgrajena na industrijskih odpadkih, iz katerih se danes v reko Hudinjo izločajo težke kovine. V Mežiški dolini moramo zaradi rudnika svinca kontinuirano izvajati nadzor nad vsebnostjo svinca v krvi otrok, saj je tam narava že tako onesnažena s svincem, da lahko pride do povišanega vnosa svinca že s prehranjevanjem z domačo zelenjavo. Malo bolje jo je odneslo odlagališče rdečega blata iz predelave aluminija v Kidričevem, tam je na prizadetem okolišu danes fotovoltaična elektrarna.
Zadnja leta so pri nas žrtvovana področja predvsem odlagališča odpadkov in zbiralinice oz. predelovalnice odpadkov. Žal v zbiralnicah prihaja do požarov, dim pa razsaja po bližnji in daljnji okolici. Veste, kaj je tudi gorljiv nevarni odpadek, ki se zbira tam? Izrabljeno motorno olje. Tega v tem filmu ne boste slišali.
Tudi nafta pušča za sabo žrtvovana področja, od razlitij (razlitje tankerja Exxon Valdez na Aljaski, popustitev ventila na globokomorski naftni vrtini Deepwater Horizon v Mehiškem zalivu), onesnaženja zraka (akutno npr. Zalivska vojna, kronično vsako večje mesto). Slika spodaj kaže, kako izgleda žrtvovano področje v osrčju Teksasa zaradi črpališč nafte in zemeljskega plina, vsaka bela pikica je vrtina. Tudi tega v tem filmu ne boste videli.
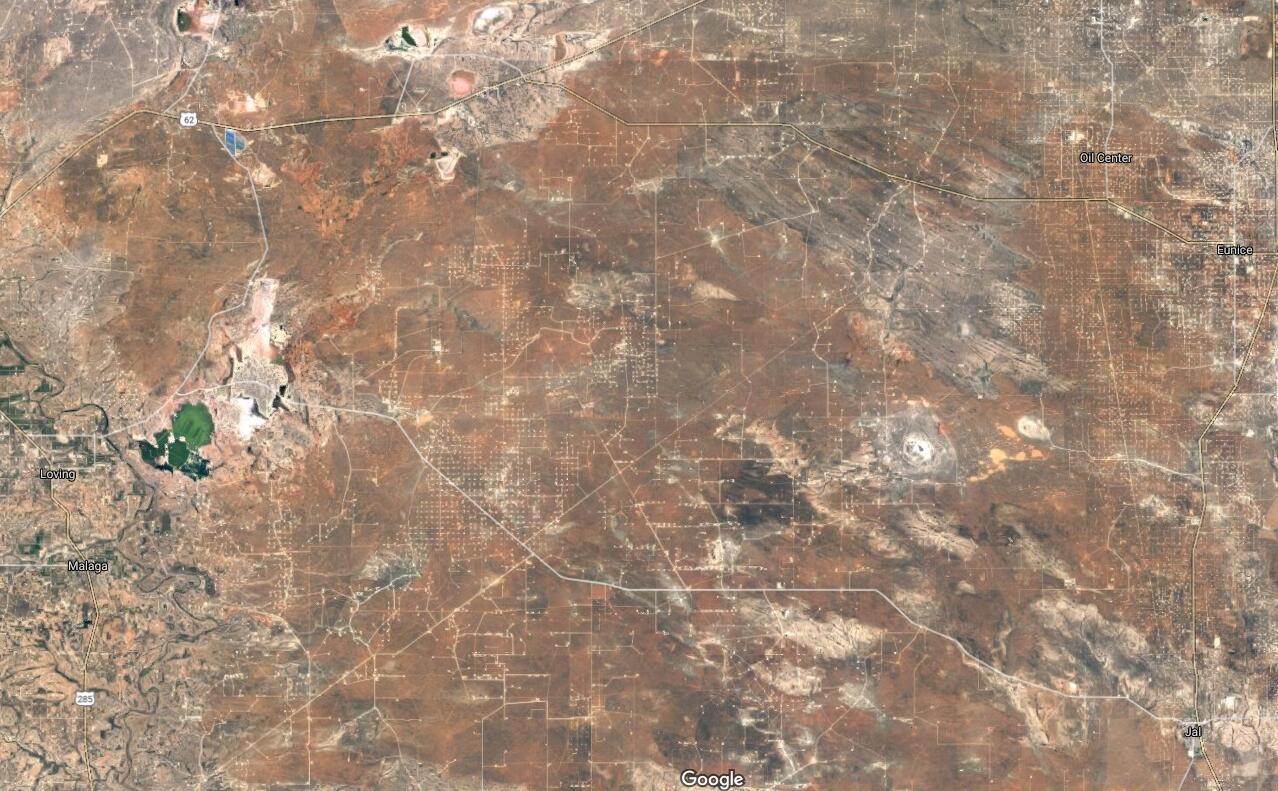
Žrtvovana področja pa puščajo za sabo tudi drugi rudniki, kjer človeštvo rudari baker, svinec, in vse ostale minerale. Praktično ves material, iz česar izdelujemo stvari, je prišel bodisi iz rudarjenja, bodisi smo ga pridobili iz rastlin.
Na koncu filma nekdo pozove še k spremembi celotnega družbenega sistema v smeri znižanja surovinske in energijske intenzivnosti sodobne družbe. Se strinjam, idealno bi bilo, če bi vsi kolesarili, ker je to energetsko optimalen način za prevoz oseb in za izdelavo kolesa porabimo zelo malo surovin, a močno dvomim, da bodo ljudje iz Mosta na Soči kolesarili v službo do Idrije.
Ta "dokumentarec" je predvsem širjenje strahu pred obnovljivimi viri energije, saj si za tarčo izbere zgolj sončne in vetrne elektrarne ter elektrifikacijo vozil. Da bi ta film zares bil dokumentarec, bi moral obravnavati industrijsko aktivnost na splošno, ter tudi industrijski aktivnosti na splošno pripisovati negativne okoljske učinke, po možnosti z drugačno in manj čustveno nabito besedno zvezo kot "temne strani".
Negativne okoljske učinke, degradacijo okolja in zastrupljanje povzročajo praktično vsa naša dejanja. "Zelena energija" oz. pravilno rečeno obnovljivi viri energije imajo za spremembo tudi potencialno svetlo stran, če do njih premišljeno pristopimo. In pravilen pristop seveda pomeni tudi reciklažo izrabljenih produktov, kar pa seveda velja za vse materiale, ki jih uporabljamo.
Pogosto se kot argument proti sončnim elektrarnam pojavljajo subvencije. Subvencije, ki jih opisujem v tej objavi, se pojavijo na položnici vsakega plačilnega mesta pod postavko "Prispevek za SPTE in OVE". Ta izplačila nimajo nobene povezave z subvencijo, ki jo prejmejo domače sončne elektrarne. Te subvencije predstavljajo tudi največje finančno breme in so bile večinoma podeljene med leti 2009 in 2013.
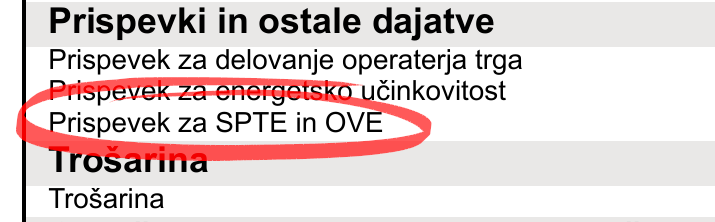
Subvencijska shema je med 2009 in 2013 delovala večinoma po pogodbi o odkupu električne energije po zagotovljeni ceni, pogodba pa je bila sklenjena za 15 let. Na začetku je ta shema imela smisel, saj so si investitorji s tem zagotovili povračilo investicije, ki sicer ne bi bila finančno smiselna. Slovenija je tako dobila znatno količino sončnih elektrarn, vendar pa se je ta shema končala relativno kmalu za tem, ko je nekdo naredil preračun koliko to stane.
Subvencioniran odkup električne energije po tej shemi izvaja Borzen oz. njegov organizacijski del Center za podpore proizvodnji zelene energije. Ta objavlja tudi poročila o izplačilih subvencij. Spodnji graf prikazuje izplačila.
Na grafu so subvencije različnim tipom elektrarn, največji znesek pa poberejo sončne elektrarne, ki so leta 2020 prejele 51% izplačil. Izplačila sončnim elektrarnam so dosegla plato okrog 70 milijonov evrov in to po tem, ko je nekdo ugotovil, da bo znesek skozi 15 letno dobo odplačevanja relativno visok. Ocena končne vrednosti subvencij za sončne elektrarne prek centra podpor bo okrog 1.050 milijonov evrov, če predvidimo poenostavljen izračun 70 milijonov krat 15 let. Do sedaj smo do vključno 3. kvartala 2021 izplačali 663 milijonov evrov, torej smo malo čez polovico. Malo čez polovico smo tudi po tem, da so subvencije dosegle plato leta 2013, danes pa smo 8 let kasneje. Te subvencije bomo v večjem znesku odplačevali še približno do 2028, potem pa bodo upadle, vsaj te iz naslova sončnih elektrarn.
Kako so te subvencije narasle na tak znesek?
Leta 2009 smo po vzoru drugih držav uvedli subvencije na obnovljive vire energije. Zagotovljena cena odkupa megavatne ure električne energije iz sončnih celic je bila leta 2009 sprva 415 EUR/MWh, potem pa je bilo predvideno, da se bo vsako leto znižala za dodatnih 7% glede na prvo leto. Med letom 2009 in 2013 se je cena celic znatno zniževala in tako se je vsako leto sprejemalo nove uredbe, ki so odkupno ceno za leto 2013 zniževale iz prvotno predvidenih 300 EUR/MWh najprej na 249 EUR/MWh, potem 216 EUR/MWh, potem na 164 EUR/MWh in končno na 150 EUR/MWh. Verjetno "najdražja" napaka je bila, da je bil samodejno priznan fiksen, v uredbi vnaprej določen strošek na enoto proizvedene električne energije, ne glede na to koliko je investicija dejansko stala.
Od takrat se je marsikaj spremenilo glede podpor, ki jih izplačuje Center. Investitorji morajo sedaj ponuditi svojo ceno, pod katero so pripravljeni prodajati električno energijo, to pa lahko center sprejme ali zavrne, tako da ne prihaja več do pomembne razlike med priznano ceno in stroški, ki jih ima investitor.
V Sloveniji smo v dobi med 2009 in 2013 dobili okrog 250MW nameščenih sončnih elektrarn. Takrat je bila cena postavitve sončnih celic visoka, zato je večji del Evrope podprl subvencijske sheme, ki niso bile neposredno odvisne od stroškov postavitve. Morda so k znižanju stroškov postavitve sončnih celic pomagale tudi te subvencije, koliko je to res, bo težko dokazati. Dejstvo pa ostaja, da so sončne celice desetletje pozneje ena ekonomsko cenejših oblik pridobivanja električne energije.
Takratno znižanje subvencij ob koncu 2013 je precej oklestilo investicije v sončne elektrarne in potem dolga leta nismo dodajali večjih količin sončnih elektrarn. To se dogaja šele zadnja leta, delno zaradi tega, ker so domače sončne elektrarne postale cenovno dostopnejše in z net-metering shemo same pokrijejo svojo investicijo, delno podjetja iščejo načine, da si znižajo izdatke za energijo oz. da zmanjšajo svoj ogljični odtis, pojavljajo pa se tudi že večje sončne elektrarne, ki jih postavljajo elektro energetska podjetja. K temu pa pomaga, da so tudi Slovenijo dosegle "evropske" višje cene električne energije.
V Londonu je že pred časom stopila v veljavo nova ureditev, kjer se je precej razširil obseg ultra-nizko-emisijske cone, kamor smejo brez plačila dodatne okoljske globe vstopiti zgolj vozila, ki delajo manj izpustov.
Slovenija take ureditve niti ne omogoča v zakonodaji, tako da v Ljubljano lahko mirno zapelje vsako vozilo, ne glede na to koliko emisij dela. Posebej problematični so starejši težki tovornjaki, ki ne dosegajo niti emisijskega standarda EURO 3.
Ljubljana, ki ji župan pravi "najlepše mesto na svetu", se je po kvaliteti zraka na lestvici 323 evropskih mest uvrstila na 260. mesto. Precej slabo!
Ljubljana ima Odlok o načrtu za kakovost zraka na območju Mestne občine Ljubljana, v tem si lahko v prilogi 2 preberemo podrobnejši seznam ukrepov. Med ukrepi na prometnem področju so tudi omejevanje hitrosti na avtocestah in hitrih cestah na območjih s slabo kakovostjo zraka, kadar agencija razglasi njegovo čezmerno onesnaženost (točka 4.2.3) in prepoved vožnje tovornih vozil na severni ljubljanski obvoznici (točka 4.2.4). Naveden je tudi nadzor nad izpusti iz vozil s čezmernimi emisijami (točka 4.2.5).
Emisijski standard Euro I je bil sprejet leta 1992, pred 29 leti, in za tovorna vozila ne predvideva nobenih omejitev glede količine izpustov prašnih delcev. Prve znatne izboljšave je prinesel šele standard Euro III, v veljavi od 1999, kar je kar 22 let nazaj. Kljub temu lahko ta 20, 30 ali več let stara tovorna vozila prosto vozijo po mestnih ulicah in onesnažujejo zrak, ob taki starosti pa je vprašljivo tudi njihovo vzdrževanje oz. obrabljenost motorja, kar izpuste le še povečuje.
Za primerjavo si lahko na spletni strani DARS prek interaktivnega kalkulatorja izračunamo koliko informativno stane cestnina za tovorna vozila. Za lažji preračun vzamemo odsek med priključkom Logatec in Celje zahod, razdalja med njima je 100,29km.
Emisijski razred | Število osi | Cestninski razred | Cestnina Logatec – Celje Z. (100,29km) |
Pribitek |
---|---|---|---|---|
E0 | 2 | R2 | 24,00 EUR | 9,07 EUR |
E6 | 2 | R2 | 14,93 EUR | |
E0 | 3 | R3 | 26,66 EUR | 10,07 EUR |
E6 | 3 | R3 | 16,59 EUR | |
E0 | 4+ | R4 | 55,09 EUR | 20,96 EUR |
E6 | 4+ | R4 | 34,13 EUR |
Pozorno oko lahko opazi, da je cestnina na avtocestah za vozila emisijskega razreda E0 znatno dražja od vozil emisijskega razreda E6. Kar pomeni, da DARS s cestnino na avtocestah na nek način bolj spodbuja rabo okolju in človeku prijaznejših tovornih vozil kot to počne MOL ali katera koli druga občina.
Energetika Ljubljana je predzadnji vikend letošnjega oktobra opozorila svoje odjemalce, da lahko pride do izpada dobave toplote v večjem delu severne Ljubljane. Razlog? Zahtevni poseg pri gradnji nove plinsko-parne enote. Energetika Ljubljana namreč menja stara premogovna kotla, blok 1 in blok 2, ki dovajata toploto po Ljubljani, s plinsko parno enoto, ki bo poleg toplote proizvajala tudi električno energijo.
Plinske elektrarne so lahko večje, kot so te v Termoelektrarni-toplarni Ljubljana ali blok 6 in 7 v Brestanici, lahko pa so tudi manjše, kot so npr. enote za kogeneracijo, ki se nameščajo v večje zgradbe, ki potrebujejo večje količine toplote, npr. šole, domove starejših občanov ali hotele.
Večje elektrarne so praviloma turbinske in lahko kot nadomestno gorivo uporabljajo tudi dizel oz. kurilno olje. Manjše so pogosto batni motor na notranje izgorevanje in ponavadi delujejo samo na plin.
Koliko stane postavitev plinske elektrarne?
Elektrarna | Vrsta | Električna moč (kW el) | Toplotna moč (kW th) | Cena izgradnje (EUR) | Cena EUR/kW el moči | Zgrajena |
---|---|---|---|---|---|---|
TEB Blok 6 | Turbina | 53.000 | - | 35.000.000 € | 660 €/kW | 2018 |
TEB Blok 7 | Turbina | 56.000 | - | 26.450.000 € | 472 €/kW | 2021 |
TEB6 in TEB7 | Turbina | 109.000 | - | 129.548.221 € | 563 €/kW | 2021 |
TETOL Plinsko parna enota 1 | Turbina | 139.000 | 118.000 | 129.548.221 € | 832 €/kW | 2021 |
Toplarna Šiška (1998) | Turbina | 6.000 | ? | 1,2 milijarde SIT (1998) | ? €/kW | 1998 |
Toplarna Šiška (2022) | Turbina | 7.520 | ? | 9.680.743 € | 1287 €/kW | 2022 |
Dijaški dom Novo Mesto | Batni motor | 50 | 80 | 110.000 € | 2200 €/kW | 2010 |
Gostišče Julči | Batni motor | 5,5 | 12,5 | 23.000 € | 4182 €/kW | 2010 |
V tej tabeli je sicer kar nekaj neprimerljivih kategorij, saj sta Termoelektrarna Brestanica blok 6 in 7 enostaven cikel, plinsko parna enota TETOL je kombiniran cikel, zraven pa sta še dva primera kogeneracije na plin, ki sta precej manjša.
Termoelektrarna Brestanica je postavila dva nova bloka, ki ju je potrebno upoštevati skupaj, ker je bilo v okviru bloka 6 izvedenih tudi nekaj del za blok 7. Elektrarni sta enostavnega cikla, kar pomeni da je njun izkoristek tam v tridesetih odstotkih, ker pa delujeta na plin, sta med dražjimi elektrarnami po strošku na kWh električne energije. Cena za postavitev večje elektrarne na plin z enostavnim ciklom je približno 563 EUR na kW električne moči.
Plinsko-parna enota termoelektrarne in toplarne Ljubljana izkorišča, kot že ime pove, plin in paro. Ima dve turbini Siemens SGT-800, ki delujeta na plin, njun izpuh pa bo grel vodo za parno turbino, tako da bo izkoristek precej boljši. Proizvajalec navaja, da lahko te turbine dosegajo izkoristke do 58%, kar pomeni precej manjši, skoraj polovični strošek za proizvodnjo energije. Cena postavitve večje elektrarne na plin s kombiniranim ciklom je približno 832 EUR na kW električne moči, se pa je potrebno zavedati, da toplarna koristno izkorišča tudi toploto.
V letu 2022 bo zamenjana tudi plinska turbina v toplarni Šiška, ki je bila postavljena leta 1998, kar daje neko oceno 23 let življenjske dobe takega stroja. Vedeti je tudi treba, da toplarna v Ljubljani deluje večino leta.
Tretja kategorija so manjše naprave za kogeneracijo, ki so običajno večji ali manjši batni motorji na notranje izgorevanje, ki za gorivo uporabljajo plin, iz izpušnih plinov pa se pridobiva toplota za ogrevanje ali sanitarno toplo vodo. Te naprave so manjše in zato precej dražje in so bile bolj odvisne od subvencij, tako je njihova vgradnja po letu 2015, ko se je spremenil sistem subvencij, precej zamrla, in nisem uspel najti javno objavljenih podatkov o ceni sveže izvedenih projektov. Poglaviten kriterij pri vgradnji tovrstnih naprav pa je vselej potreba po toploti - če je večji del leta dovolj velik odjem toplote, potem je smiselno pogledati tudi kogeneracijo. 50kWel SPTE napravo je leta 2010 Dijaški dom Novo mesto postavil za ceno 2200 EUR na kW električne moči.
Vse te naprave pa so seveda šele začetek 20-letne naročnine na energent - plin, ki je zaenkrat še vedno fosilno gorivo.
- Viri:
- Najava motene ali prekinjene dobave toplote v Ljubljani (novica Energetike Ljubljana)
- TEB 6 gradijo brez izdelane vloge v sistemu (delo.si)
- Projekt Blok 7 v TeB se nadaljuje, v letu 2019 nad načrti (eposavje.com)
- Letak o plinsko parni enoti toplarne Ljubljana (energetika.si, PDF)
- Letno poročilo Energetike Ljubljana za leto 2020 (energetika.si, PDF)
- Siemens SGT-800 (siemens.com, tehnični podatki proizvajalca plinske turbine)
- Nova enota za soproizvodnjo toplotne in električne energije (ljubljana.si, o toplarni Šiška)
- Pregled sistemov soproizvodnje toplote in električne energije z izbranimi primeri iz Evrope (energetika-portal.si, o toplarni Šiška, PDF)
- Cogeneration case studies handbook, Code project (informacije o Dijaškem domu Novo mesto, PDF)
- Soproizvodnja toplote in električne energije (SPTE) (Oglas na finance.si, o Gostišču Julči)